About
The fourth shared task for Translation Inference Across Dictionaries (TIAD 2021) is aimed at exploring methods and techniques for automatically generating new bilingual (and multilingual) dictionaries from existing ones in the context of a coherent experiment framework that enables reliable validation of results and solid comparison of the processes used. This initiative also aims to enhance further research on the topic of inferring translations across languages.
TIAD 2021 will be held in conjunction to the 3rd Conference on Language, Data and Knowledge (LDK 2021) in Zaragoza (Spain) on September 1, 2021.
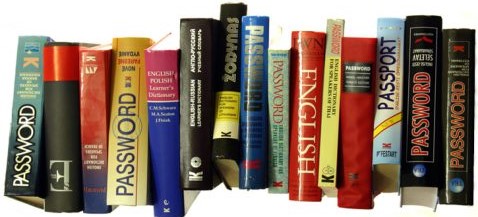
Task definition
The objective of TIAD shared task is to explore and compare methods and techniques that infer translations indirectly between language pairs, based on other bilingual resources. Such techniques would help in auto-generating new bilingual and multilingual dictionaries based on existing ones.
In particular, the participating systems will be asked to generate new translations automatically among three languages, English, French, Portuguese, based on known translations contained in the Apertium RDF graph. As these languages (EN, FR, PT) are not directly connected in this graph, no translations can be obtained directly among them there. Based on the available RDF data, the participants will apply their methodologies to derive translations, mediated by any other language in the graph, between the pairs EN/FR, FR/PT and PT/EN.
Participants may also make use of other freely available sources of background knowledge (e.g. lexical linked open data and parallel corpora) to improve performance, as long as no direct translation among the target language pairs is applied.
Evaluation of the results will be carried out by the organisers against manually compiled pairs of K Dictionaries and other resources.
Other language pairs and evaluation data might be included in the evaluation process by the organisers, in which case the participants will be conveniently informed.
Publication of results
Participants will submit a system paper that should include a description of the system, the way the data have been processed, the applied algorithms, the obtained results, as well as the conclusions and ideas for future improvements. The papers will be peer reviewed prior to publication to confirm that all aspects are well covered.
The workshop will accept also regular papers from participants who are not participating in the shared task but still have worked in the topic of translation inference and want to publish novel results or ideas, maybe with different datasets and experimental basis as the ones proposed in this shared task. Such papers will be peer reviewed on the basis of their scientific quality.
Both types of papers should have 6-8 pages and be formatted according to LNCS guidelines and will be submitted through EasyChair. All the accepted papers will be published as part of the TIAD proceedings and presented during the workshop.
UPDATE: TIAD-21 proceedings are already available at http://ceur-ws.org/Vol-3064/
How to participate in the shared task
1. Contact us so we can be aware of your participation and inform you about any possible change, issue, etc. (see contact details at the bottom of this page)2. Read the task and data description
3. Get the input data (initial translations)
4. Run your system on the input data
5. Get the output results (inferred translations) and format it according to the guidelines (see the task and data description section)
6. Send the output data to the organisers and wait for the evaluation results
7. Write and submit a system description paper
8. Present your paper at the workshop
Important dates (updated!)
01/02/2021 - Technical description of evaluation process and
data provided by the organisers
23/04/2021 - Submission of regular papers (not participating
systems)
14/05/2021 - Submission of results by participating systems /
notification of regular papers
14/06/2021 - Evaluation results communicated by organisers /
camera–ready of regular papers
09/07/2021 - Submission of system description papers
01/09/2021 - TIAD 2021 workshop day
Organisers
- Jorge Gracia, University of Zaragoza, Spain
- Besim Kabashi, Friedrich-Alexander University of Erlangen-Nuremberg and Ludwig-Maximilian University of Munich, Germany
- Ilan Kernerman, K Dictionaries – Lexicala, Tel Aviv, Israel
- Noam Ordan, University of Haifa, Israel
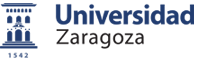

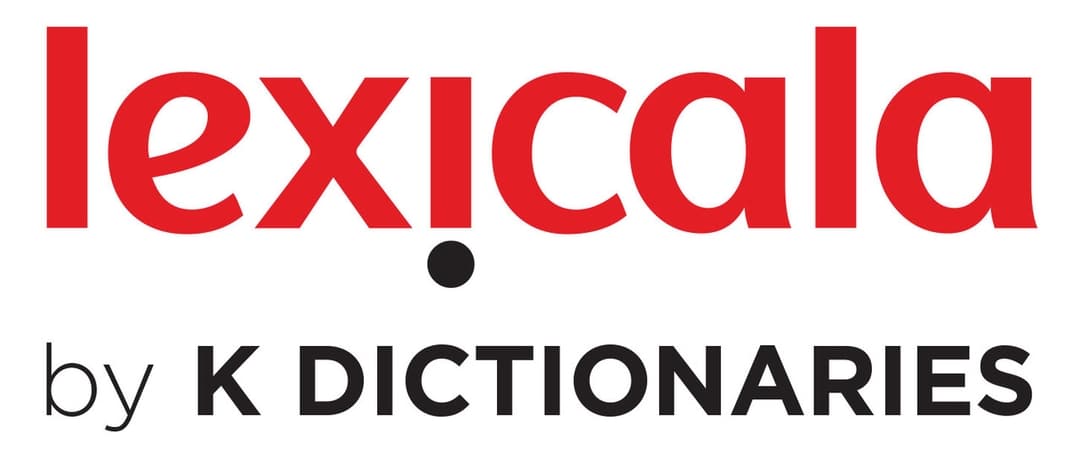
Previous editions
Reviewing committee
- Omri Abend, Hebrew University of Jerusalem, Israel
- Sina Ahmadi, National University of Ireland Galway, Ireland
- Julia Bosque-Gil, University of Zaragoza, Spain
- Thierry Declerck, DFKI, Germany
- Jorge Gracia, University of Zaragoza, Spain
- Dagmar Gromann, University of Vienna, Austria
- Yinxia Huang, PaiChai University, South Korea
- Besim Kabashi, Friedrich-Alexander-Universität Erlangen-Nürnberg, Germany
- Ilan Kernerman, K Dictionaries – Lexicala, Israel
- Fahad Khan, Istituto di Linguistica Computazionale "Antonio Zampolli", Italy
- Simon Krek, Jožef Stefan Institute, Slovenia
- Maite Melero, Barcelona Supercomputing Center, Spain
- Elena Montiel-Ponsoda, Universidad Politécnica de Madrid, Spain
- Noam Ordan, University of Haifa, Israel
- Georg Rehm, DFKI, Germany
- Artem Revenko, Semantic Web Company, Austria
- Arvi Tavast, Estonian Language Institute, Estonia
- Andrzej Zydroń, XTM International, UK
References
Some papers describing previous work on translation inference across dictionaries:- I. Kernerman, S. Krek, J. P. McCrae, J. Gracia, S. Ahmadi, and B. Kabashi (Eds.), Proceedings of Globalex 2020 Workshop on Linked Lexicography. ELRA, 2020. See https://www.aclweb.org/anthology/volumes/2020.globalex-1/
- Gracia J., Kabashi, B., Kernerman, I. (Eds.): Proceedings of "TIAD-2019 Shared Task – Translation Inference Across Dictionaries" co-located with the 2nd Language, Data and Knowledge Conference (LDK 2019). Leipzig, Germany, May 20, 2019. See http://ceur-ws.org/Vol-2493/.
- Gracia J., Kabashi, B., Kernerman, I., Lanau-Coronas M., Lonke D.: Results of the Translation Inference Across Dictionaries 2019 Shared Task. In Proceedings of TIAD-2019 at LDK 2019, Leipzig, Germany, May 20, 2019. See http://ceur-ws.org/Vol-2493/summary.pdf
- McCrae J. P., Bond, F., Buitelaar, P., Cimiano, Ph., Declerck, Th., Gracia, J., Kernerman, I., Montiel Ponsoda, E., Ordan, N. and Piasecki, M. (Eds.): Proceedings of the Workshop “Shared Task on Translation Inference Across Dictionaries”, co-located with the 1st Conference on Language, Data and Knowledge (LDK 2017). Galway, Ireland 2017. See http://ceur-ws.org/Vol-1899/.
- Villegas, M., Melero, M., Gracia, J., and Bel, N. 2016. Leveraging RDF Graphs for Crossing Multiple Bilingual Dictionaries. In LREC 2016 Proceedings: 613–622. http://repository.dlsi.ua.es/242/1/pdf/175_paper.pdf
- Saralegi, X., Manterola, I. and San Vicente, I. 2011. Analyzing Methods for Improving Precision of Pivot Based Bilingual Dictionaries. In Proceedings of the Conference on Empirical Methods in Natural Language Processing, 846–856. ACL. http://dl.acm.org/citation.cfm?id=2145526.
- Shezaf, D. and Rappoport, A. 2010. Bilingual Lexicon Generation Using Non-Aligned Signatures. In Proceedings of the 48th Annual Meeting of the Association for Computational Linguistics, 98–107. ACL. http://dl.acm.org/citation.cfm?id=1858692
- Kaji, H., Tamamura, S. and Erdenebat, D. 2008. Automatic Construction of a Japanese-Chinese Dictionary via English. In LREC 2008 Proceedings: 699–706.
- Mausam, Soderland, S., Etzioni, O,, Weld, D, Skinner, M. and Bilmes, J. 2008. Compiling a Massive, Multilingual Dictionary via Probabilistic Inference. In Annual Meeting of the Association of Computational Linguistics. ACL. https://www.cs.washington.edu/sites/default/files/ai/papers/tmpiVvJEg.pdf
- Tanaka, K. and Umemura, K. 1994. Construction of a Bilingual Dictionary Intermediated by a Third Language. In Proceedings of the 15th Conference on Computational Linguistics, Volume 1, 297–303. ACL. http://dl.acm.org/citation.cfm?id=991937
Supported by
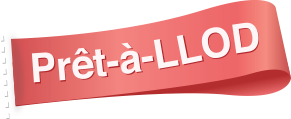
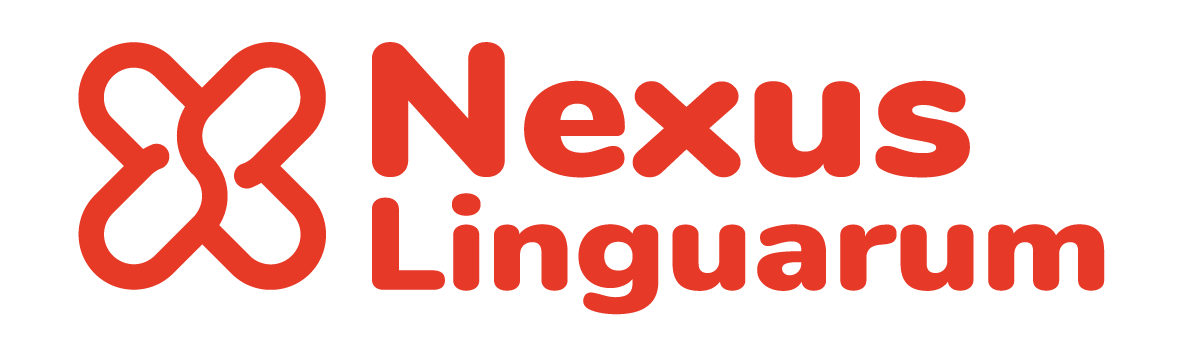
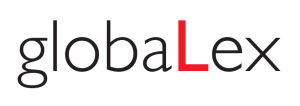
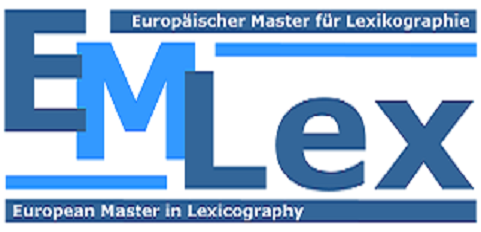
NexusLinguarum and Prêt-à-LLOD have received funding from the Horizon 2020 European Union (EU) Research and Innovation programme.
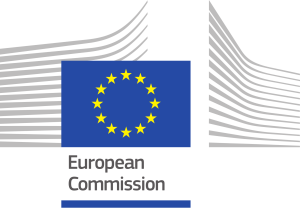
Contact
To inquire about any aspect of this shared task please send an email to Jorge Gracia